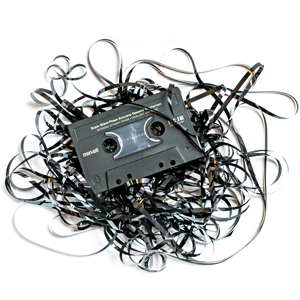
In 2025, I’m focusing on error rates and data quality. My plan is to update this post through the year.
Martin Jordan reminded me about error rates
In August 2024, Martin Jordan (Head of Design and User Research for the German government’s Digital Service) posted on social media about error rates:
Non-fun fact:
There are error rates for various government services and related forms far beyond 90%.
Government does not ask people to submit the necessary information and documents and then cannot make a decision.
Error rate is now a central metric tracked in various of our work streams.
I was delighted to see “error rate” as a central metric.
Many years ago, I did some conference talks about understanding the costs of data capture that included some discussion of types of errors and their effects on costs, but it’s been a long while since I revisited the topic – apart from regularly finding out (and telling anyone who would listen) that error rates of at least 100% (every form has at least one mistake) are highly typical on any complex form that has not had recent, effective, user-centred design intervention. It’s not at all unusual for the more complex government services to have error rates of 600%: each user taking on average six (6!) attempts to get through.
And yet, I see very little discussion of error rates specifically or data quality in general. The UK Government Service Manual requires services to publish four metrics:
but there is nothing there about error rates.
The Service Manual urges services to define their own performance metrics, but none of the examples discuss error rates or data quality and I have not (yet) been able to find any published metrics for UK government services more recent than 2021, when the UK Government Digital service decided to retire the Performance Platform.
Good quality data is crucial for AI
Some of us are enthusiastic adopters of the assorted technologies that now get lumped together under the heading of Artificial Intelligence (AI). Others are cautious experimenters. There are many who fight AI tooth and nail as we increasingly resent the way that we are forced into AI-mediated experiences, despite our many reservations, and worry about the implications for marginalised communities and the climate emergency. Some of us veer between all these views, depending on the context and the specifics of each application.
But I think all of us can easily agree: if we feed poor quality data into AI, we can be assured that we’ll get poor quality results out of it. And yet, high error rates are very likely to contribute to poor data quality.
As we barrel towards AI everywhere, whether we like it or not, it seems to me to add a level of urgency to my interest in error rates and to their effect on data quality. So I decided that 2025 would be a good year to brush off my ideas, and try to learn as much as possible about what’s currently happening in the world of government services around error rates and data quality.
I led a discussion about error rates and data quality at GovCamp
To get a first pass on the concepts, I pitched a session that attracted quite a large audience at the January 2025 GovCamp unconference. You can read the notes from the wide-ranging discussion, and I’d like to highlight three points that stood out for me:
- One person shared an anecdote for a government service that claimed “We don’t have a fraud problem because we don’t measure it”. (This particular service hits the headlines several times a year because of some scandal or other involving fraud).
- We discussed the value of metadata – the data that tells us what’s in the data.
- I learned about the UK Government Data Quality framework, which seemed well worth further investigation.
This discussion really encouraged me in my plan to focus on error rates and data quality in 2025, and I’m grateful to everyone who shared their time and thoughts.
A team at HMRC inspired a five-aspect framework for errors
In February, I had a really useful discussion at HMRC about the types of error rates we encounter. Together, we developed a five-aspect framework for errors. We agreed that most of our experience has been in the area of discovering and fixing “problems along the way” – the errors typically detected when someone tries to put an answer into a form that fails some sort of validation.
We did not have time to think very deeply about error rates, which consider the errors in proportion to the number of forms submitted. Or maybe, to the number of users who try to do a form. Or maybe, the number of people who ought to do the form – of whom, only a proportion actually do it.
Content Club attendees contributed some more research for me
I was very happy that UK Cross-Government Content Club invited me to speak at what turned out to be their very first meeting. They were kind enough to accept my suggestion of “Error messages and error rates” as my topic, and I asked attendees to do a little preparation by spending no more that 15 minutes finding out what, if anything, the service they were working on did to track their error rates.
As I write this, in April 2025, I’m still thinking about the points that they shared.
I’ll be developing and testing this theme at events over the spring and summer
I’m looking forward to testing out ideas on error rates and data quality, and collecting feedback, at two more events:
- Agile Manchester – Do you know your error rates? in mid-May
- UX Connect: Garbage in, Garbage Out – Measuring error rates & data quality to get ready for AI in Aarhus, Denmark in June.
I plan to report on what I learn in this post, and overall hoping to glean some ideas about how we can measure error rates and improve data quality.
In the meantime, I’m keen to hear from any colleagues who are already measuring error rates, or looking at how to improve data quality.